Reservoir Blog
- Home
- Reservoir Blog
- How well do you know your data?
How well do you know your data?
«Start with the data, assess its quality, and, as a team, integrate it.»
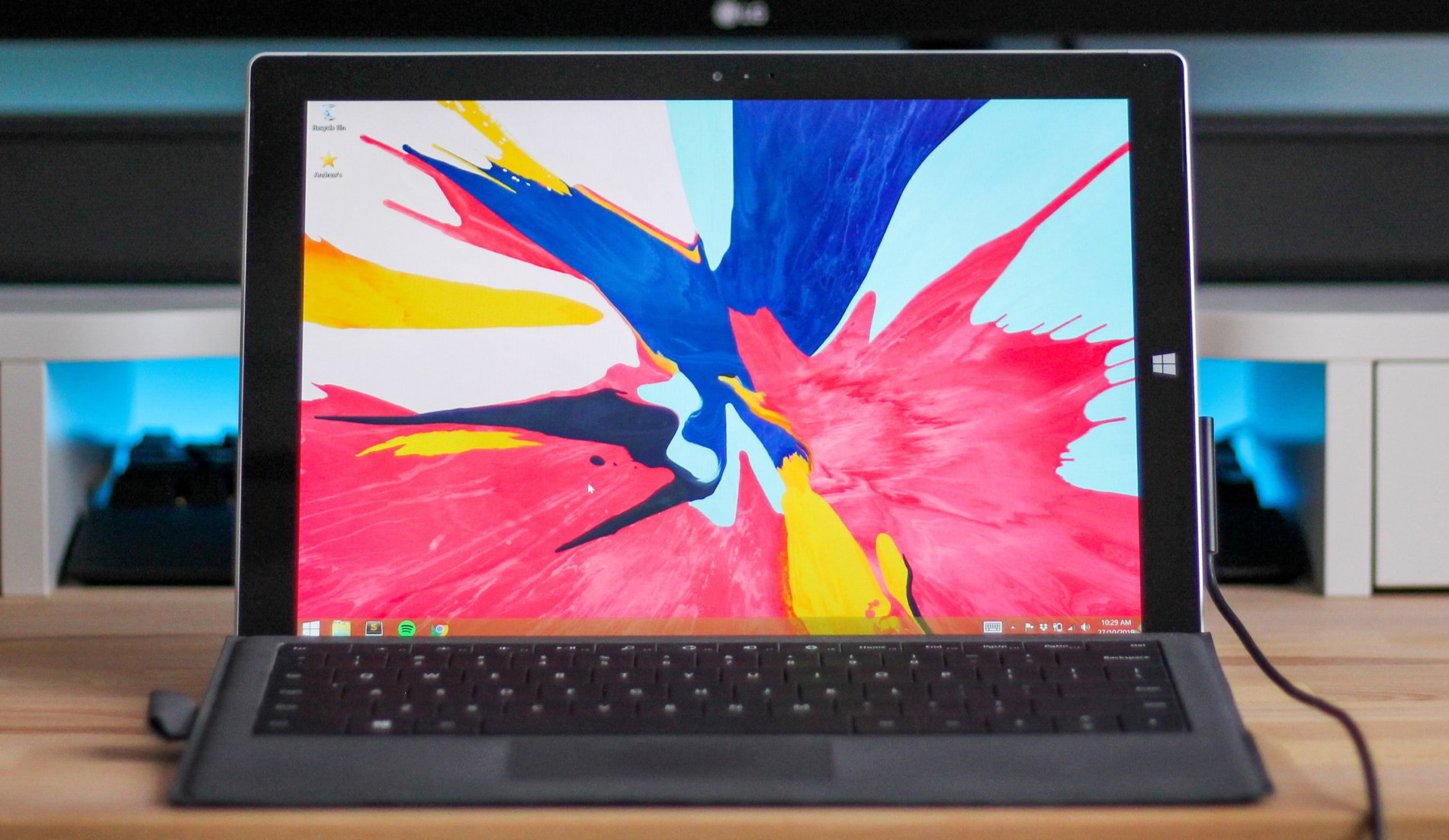
I have been involved in many reservoir modelling projects. They were all different, not only in terms of reservoir characteristics, but also in terms of methodologies.
In this article, I would like to share some tips, based on my experience, on how to deal with the data typically gathered during the life of a field, and how to maximise its value for integrated reservoir studies. My objective is to help you ask yourselves, and others, the right questions when dealing with data.
I will stress the importance of “curiosity” and team work. We will look at several examples of data uncertainties that subsurface teams need to be aware of during their modelling and simulation projects. We will focus on data that is matched during history matching: well rates, pressures and flow profiles.
“Be curious and proactive”
I am curious. I like to know where data comes from. I like to know its origin, its history. It’s not that I am nosy... In fact, data quality (“the degree to which data is fit for purpose”) is variable, some processing is often required, and the validity of subsurface models will be affected by inappropriate use of data -“garbage in, garbage out”.
So, we should always try to be critical of data, its quality, and how it is used in subsurface studies. Start at the beginning and talk to the operational staff who acquires it. Be proactive and attend the planning meetings before data acquisition operations such as logging and testing in particular. Explain what data you are interested in and how you are planning to use it. Ask how the equipments work, what their limitations are, what quantities are actually measured. Those multidisciplinary discussions will help you and your team to assess data uncertainty. I have learnt a lot during such discussions, and I am sure you will too.
“Be aware of measurement accuracy, and operational and interpretation uncertainties”
Let’s now focus on some data that is typically matched during history matching: formation pressures, well production rates and pressures, production-inflow profiles. Some quantities are measured, but most are the results of calculations or interpretations.
Measurements accuracy describes the trueness of a measurement; in other words, how close it is to the real value. For data to be considered high quality, it has to be accurate, in addition to other criteria. Product data sheets usually include accuracy values. For example, a quartz pressure gauge could be reported to have an accuracy of 3 psi. I always look at the product data sheets, to compare some tools during the planning stage, and to assess the quality of data already acquired.
Data uncertainty may also be related to operational limitations. I have been involved in many debates on depth uncertainties, because it is actually a multifaceted topic! Drilling depths and wireline logging depths are different because of differences in string stretches. Wellbore surveys measurements need to be processed to obtain true vertical depths. In the end, there can be a few meters of uncertainty on true vertical depths (TVD).
Finally, measured data is seldom fit for use in models as is, and requires some processing. The processing steps - calculations, interpretations - add uncertainty to the data eventually used in models. Let’s look in more detail at the examples of production allocation and production log interpretation.
“Well production rates are back-allocated and are uncertain”
When calibrating a model, reservoir engineers try to match the historical production rates of oil, water and gas for each well. These historical rates often are the results of calculations that carry more or less uncertainty depending on each field metering configuration and strategy. If you have never visited a producing field, you may not be aware of this, so let me give more explanation.
The most accurate rates are the ones provided by fiscal metering (custody transfer) systems, with a rate uncertainty typically less than 1%. Fiscal metering systems are installed at the outlet of a production facility, where the fluids are transferred to another party. This means that the measurements correspond to the commingled production of all the wells producing into the facility.
In the absence of accurate flow metering for each well, it is necessary to back-calculate the contribution of each well to the total production: this is called “production allocation”.
Production allocation is based on occasional well test data and permanent, or daily, well pressure measurements. Well tests are used either to calibrate well performance models, or to pro-rate the contribution of each well. When well testing conditions are different from operating conditions, well performance models allow estimating well production rates as a function of their measured pressures. As for any model, some interpretation is required, and there is not just one model matching the measured data. Of course, the separator well test rates are also uncertain. In addition, field historical water rates are often less accurate than oil rates.
The resulting uncertainty in back allocated rates is around 10%. It is important to bear this in mind when history matching: matching “perfectly” quantities with that level of uncertainty would incorrectly reduce the range of acceptable models.
“Production log interpretation is uncertain, but even qualitative data may be integrated in reservoir characterisation”
I always ask for more production logs! While production allocation allows splitting the total field production between wells, a production log can help split well production between reservoir zones: this is called “production flow profiling”. It can show reservoir heterogeneities that were not suspected, and I have seen high permeability streaks on production logs on many occasions. Production logs may also be run to monitor fluid contacts movements, or detect flow behind casing. This is great to identify well intervention options.
Operationally, production logging for flow profiling includes a spinner, whose rotation speed is converted to fluid velocity after calibration. Fluid density measurements allow distinguishing gas and liquids. More probes may be included to estimate the fractions of each fluid (“holdups”).
Those types of production logs are not run as widely as openhole logs. Their interpretation is therefore less routine. Yet, it involves many uncertainties – spinner calibration, PVT, slip velocities and holdups - and several solutions should be tested.
In some cases, it may be decided to use the production log interpretation results qualitatively rather than quantitatively for history matching. Qualitatively, production logs can highlight flow units and permeability streaks, and this should be integrated in the reservoir characterisation constructed by the subsurface team.
Work as a team, and embrace uncertainty
In summary, we have seen that, beyond the relative lack of data compared to the extent of a field, data is uncertain due to measurement inaccuracies, operational limitations, and non-uniqueness of interpretations.
It is important for all members of a subsurface team to be critical of data. I am convinced that a multi-disciplinary approach, involving operational staff, geoscientists and engineers helps to understand the origin, quality, and uncertainties of data and to integrate it properly in models and simulation projects.
Reservoir engineers need to know that the data they match during history matching is often a result of calculations and interpretations, which adds some degree of uncertainty. As a young engineer, I tried to match “perfectly”, but that’s not the right thing to do! The level of uncertainty in the historical data, especially well rates, needs to be taken into account when history matching: overfitting historical data will improperly reduce the range of possible solutions.
Data may also be used as a qualitative input for reservoir characterisation, for instance to highlight flow units that are critical to dynamic behaviour.
It is one of the roles of a subsurface team to assess data quality – how fit for purpose it is - and to account for data uncertainty in reservoir modelling and simulations. My next articles will discuss reservoir model quality check, knowledge sharing and making the most of technology.
BIOGRAPHY
Claude Barthès started her career in the Oil and Gas Upstream industry in 1998. She holds a MSc in Petroleum Engineering from IFP School, France. She has worked as reservoir engineer for various companies - BP, Total, PetroSA, Woodside, Tullow - and has lived in several countries in Africa, Europe, South America and Asia. She is now based in France and works with Amarile.
Credit photo : Andrew Mantarro